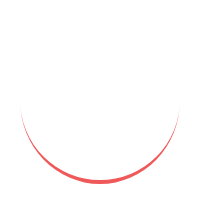
DFSET (Dynamic Feature Selection and Extraction Technique) is a cutting-edge approach in data analysis and machine learning that revolutionizes the way we extract relevant features from complex datasets. In this article, we will explore the concept of DFSET, its benefits, implementation strategies, and future prospects. Whether you are a data scientist, business owner, or tech enthusiast, understanding DFSET can provide valuable insights for improving decision-making processes and achieving better outcomes.
1. What is DFSET?
DFSET is a sophisticated methodology that aims to optimize feature selection and extraction in data analysis. It utilizes advanced algorithms and statistical techniques to identify the most relevant and informative features from large datasets. By reducing the dimensionality of the data while preserving its inherent characteristics, DFSET enhances the accuracy, efficiency, and interpretability of machine learning models.
2. Benefits of DFSET
DFSET offers several notable benefits for businesses and researchers:
Improved Accuracy: By focusing on the most relevant features, DFSET enhances the accuracy and predictive power of machine learning models. This leads to more reliable insights and better decision-making.
Efficient Resource Allocation: DFSET reduces the computational burden by eliminating irrelevant or redundant features. This enables efficient resource allocation, especially in resource-constrained environments.
Enhanced Interpretability: By selecting meaningful features, DFSET improves the interpretability of models, making it easier to understand the underlying factors influencing predictions.
Overcoming the Curse of Dimensionality: DFSET effectively addresses the "curse of dimensionality" problem by reducing the number of features while maintaining the integrity of the data. This leads to more efficient and reliable model training.
Time and Cost Savings: DFSET streamlines the data analysis process by reducing the time and effort required for feature selection. This translates into cost savings and accelerated insights.
3. How Does DFSET Work?
DFSET employs a multi-step approach to feature selection and extraction:
a. Data Preprocessing: The first step involves data preprocessing, including cleaning, normalization, and handling missing values. This ensures the data is in a suitable format for analysis.
b. Feature Ranking: DFSET utilizes various ranking techniques such as correlation analysis, mutual information, and statistical tests to evaluate the relevance of each feature. The features are then ranked based on their importance.
c. Feature Selection: In this step, DFSET selects the top-ranked features according to predefined criteria, such as a certain threshold of importance or a specific number of features to retain.
d. Feature Extraction: DFSET employs advanced dimensionality reduction techniques such as principal component analysis (PCA), independent component analysis (ICA), or autoencoders to extract new features that capture the most salient information from the data.
e. Model Training and Evaluation: The final step involves training a machine learning model using the selected or extracted features and evaluating its performance using appropriate metrics.
4. DFSET vs. Traditional Methods
DFSET offers significant advantages over traditional feature selection methods:
Flexibility: DFSET adapts to various types of datasets and can handle both structured and unstructured data, whereas traditional methods may be limited in their applicability.
Automatic Feature Extraction: Unlike traditional methods that rely solely on manual feature selection, DFSET incorporates automated feature extraction techniques. This enables the discovery of complex patterns and relationships that may go unnoticed with manual selection.
Improved Performance: DFSET's combination of feature selection and extraction optimizes the model's performance by focusing on the most informative features and reducing noise and redundancy.
Scalability: DFSET can handle large-scale datasets efficiently, making it suitable for big data applications. Traditional methods may struggle with computational limitations when dealing with extensive datasets.
5. Implementing DFSET in Your Business
To implement DFSET effectively, consider the following steps:
a. Understand Your Data: Gain a comprehensive understanding of your data, including its structure, characteristics, and intended use. This helps determine the most relevant features to extract or select.
b. Select the Right Techniques: Familiarize yourself with different feature ranking, selection, and extraction techniques. Choose the methods that align with your data and objectives.
c. Experiment and Evaluate: Implement DFSET on a small subset of your data and evaluate the results. Assess the impact on model performance, interpretability, and efficiency. Adjust the techniques if necessary.
d. Scale Up: Once you are confident with the results, apply DFSET to the entire dataset and integrate it into your data analysis pipeline. Monitor the performance and adapt as needed.
6. DFSET Case Studies
To highlight the effectiveness of DFSET, let's explore a couple of real-world case studies:
Case Study 1: Customer Churn Prediction
A telecom company implemented DFSET to predict customer churn. By selecting the most informative features related to customer behavior, usage patterns, and satisfaction metrics, they developed a churn prediction model with higher accuracy than traditional methods. This enabled them to proactively identify at-risk customers and implement retention strategies effectively.
Case Study 2: Fraud Detection
A financial institution utilized DFSET to improve fraud detection in credit card transactions. By extracting relevant features related to transaction patterns, customer profiles, and historical data, they achieved better fraud detection rates while minimizing false positives. This resulted in significant cost savings and enhanced security for their customers.
7. Common Challenges and Solutions
Implementing DFSET may come with some challenges. Here are a few common ones and their potential solutions:
Challenge 1: High Dimensionality
Solution: Apply dimensionality reduction techniques like PCA or feature selection algorithms to reduce the number of features while preserving the data's integrity.
Challenge 2: Data Quality Issues
Solution: Address data quality issues by cleaning the data, handling missing values, and removing outliers before applying DFSET techniques.
Challenge 3: Interpreting Complex Feature Representations
Solution: Utilize visualization techniques, such as scatter plots or heatmaps, to gain insights into the relationships between the extracted features and the target variable.
8. Best Practices for DFSET
To make the most of DFSET, consider these best practices:
Understand your data thoroughly before applying DFSET techniques.
Experiment with various feature selection and extraction methods to find the most suitable approach for your dataset and objectives.
Regularly evaluate the performance of your models and update the selected or extracted features if necessary.
Keep track of the impact of DFSET on interpretability and ensure the final models are explainable.
Stay updated with advancements in DFSET methodologies and algorithms to leverage the latest techniques.
9. Future of DFSET
The future of DFSET looks promising as data analysis and machine learning continue to advance. With the increasing volume and complexity of data, DFSET will play a crucial role in extracting meaningful insights efficiently. We can expect further developments in DFSET algorithms, integration with deep learning frameworks, and improved interpretability techniques.
Conclusion
DFSET is a game-changer in the field of data analysis and machine learning. By leveraging advanced feature selection and extraction techniques, businesses can enhance the accuracy, efficiency, and interpretability of their models. With the ability to handle large-scale datasets and automate the extraction of relevant features, DFSET empowers organizations to make better-informed decisions and achieve superior outcomes.
DFSET FAQ
Q1: Is DFSET applicable to any type of data?
A: Yes, DFSET can be applied to various types of data, including structured, unstructured, and even text or image data.
Q2: Can DFSET handle high-dimensional datasets?
A: Yes, DFSET addresses the curse of dimensionality problem by reducing the number of features while preserving the integrity of the data.
Q3: Does DFSET require expert knowledge in data analysis?
A: While some understanding of data analysis is beneficial, DFSET provides automated techniques that can be implemented by data scientists or analysts.
Q4: Can DFSET be combined with deep learning algorithms?
A: Yes, DFSET can be integrated with deep learning frameworks to enhance feature extraction and improve model performance.
Q5: Is DFSET suitable for small businesses?
A: Yes, DFSET can benefit businesses of all sizes by improving accuracy, efficiency, and interpretability in data analysis and decision-making processes.
DFSET is a diploma level course. It is the application of science and engineering principles to protect people, property, and their environments from the damaging and destructive effects of fire and smoke. The duration of this course is generally 1-year long And its syllabus is divide in 2-semester. The program start during the times of emergency to train candidates to gain knowledge about the preventive measures. The main prurpose of the course is training sessions carried on in real life scenarios as well as mock drills and the science involved in fire and firefighting. The topics related to fire prevention, engineering of fire safety, communication systems and first-aid carried on all these are cover in this program.
Job Profile: