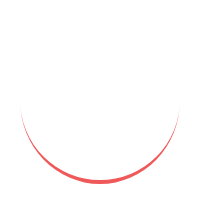
Introduction
In today's data-driven world, organizations are constantly collecting and analyzing vast amounts of data. However, without a proper framework for managing and governing this data, its potential remains untapped. This is where Data Governance (DG) steps in, providing a structured approach to managing, protecting, and leveraging data assets. In this article, we will explore the concept of DG, its benefits, implementation challenges, best practices, and future trends.
Understanding DG
What is DG?
Data Governance refers to the overall management of data within an organization. It involves defining policies, procedures, and roles to ensure data is accurate, consistent, and secure. DG encompasses data quality, data integration, data privacy, and compliance.
Importance of DG
Effective DG is crucial for organizations to harness the full value of their data. It helps establish trust in data, ensures compliance with regulations, and enables data-driven decision-making. DG also promotes collaboration and accountability, leading to improved operational efficiency.
Benefits of DG
Enhanced Data Analysis
With DG in place, organizations can rely on high-quality data for analysis. By ensuring data accuracy, consistency, and completeness, DG facilitates meaningful insights and enables data-driven strategies.
Improved Decision Making
Data governance provides a reliable and consistent framework for decision-making processes. It ensures that decisions are based on accurate and reliable data, reducing the risk of making flawed or uninformed choices.
Increased Efficiency
Efficient data governance streamlines data processes, reducing redundancy and eliminating data silos. It enables organizations to optimize their data management efforts, leading to increased operational efficiency and cost savings.
Challenges in Implementing DG
Implementing DG is not without its challenges. Organizations may face various hurdles during the process, including:
Data Quality and Integration
Poor data quality and lack of integration can hinder the effectiveness of DG. Inaccurate or incomplete data can lead to flawed insights and decisions. Organizations need robust data quality management practices to overcome this challenge.
Privacy and Security
As data governance involves handling sensitive and confidential information, privacy and security are significant concerns. Organizations must establish robust security measures and adhere to privacy regulations to protect data from unauthorized access or breaches.
Technical Infrastructure
Implementing DG requires a robust technical infrastructure capable of handling large volumes of data. Organizations need to invest in the right tools and technologies to support their data governance initiatives.
Best Practices for Successful DG
To ensure successful implementation of DG, organizations should consider the following best practices:
Clearly Define Goals
Establish clear goals and objectives for data governance. Identify key areas where DG can provide the most value and align them with the organization's overall strategy.
Establish Data Governance
Create a dedicated data governance team or committee responsible for defining and enforcing data governance policies and procedures. Assign roles and responsibilities to ensure accountability and ownership.
Implement Data Management
Develop a comprehensive data management strategy that includes data classification, data lifecycle management, and data stewardship. This strategy should encompass data acquisition, storage, integration, and archival.
Ensure Data Quality
Implement data quality management processes to ensure data accuracy, completeness, and consistency. Regularly monitor and assess data quality, and establish data quality metrics to track improvements.
Train and Educate Staff
Provide training and education programs to enhance data literacy among employees. Foster a data-driven culture and encourage staff to understand the importance of data governance in their day-to-day work.
Future Trends in DG
Data governance is an evolving discipline, and several trends are shaping its future:
AI and Machine Learning: The use of AI and machine learning technologies can automate data governance processes, improving efficiency and accuracy.
Blockchain for Data Governance: Blockchain technology offers enhanced security and immutability, making it a potential solution for data governance and integrity.
Cloud-based Data Governance: Cloud platforms provide scalability, agility, and cost-effectiveness, making them an attractive option for data governance initiatives.
Privacy and Ethics: With increasing concerns about data privacy and ethical use of data, future data governance practices will focus on ensuring transparency and compliance.
FAQs
What is the role of data governance in data analytics?
Data governance ensures that data used for analytics is accurate, consistent, and reliable. It establishes a framework for data quality, data integration, and data management, enabling meaningful and actionable insights.
How can organizations overcome data quality challenges in data governance?
Organizations can overcome data quality challenges by implementing data quality management processes, such as data profiling, cleansing, and validation. Regular monitoring and assessment of data quality are essential for continuous improvement.
Is data governance only important for large organizations?
No, data governance is important for organizations of all sizes. Regardless of their scale, organizations need to ensure data accuracy, consistency, and security to make informed decisions and maintain customer trust. How can organizations foster a data-driven culture Organizations can foster a data-driven culture by providing training and education on data literacy, encouraging data sharing and collaboration, and recognizing and rewarding data-driven initiatives and achievements.
What are some common data governance tools and technologies?
Common data governance tools and technologies include data cataloging tools, master data management systems, data quality tools, metadata management tools, and data governance frameworks.