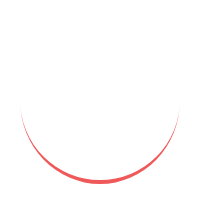
In today's digital age, the world is generating an unprecedented amount of data. Every click, purchase, and social media post contributes to this vast ocean of information. However, this data is meaningless without the right tools and techniques to extract insights from it. This is where data science, often abbreviated as DS, comes into play. DS is a multidisciplinary field that combines statistics, mathematics, programming, and domain knowledge to uncover patterns, make predictions, and solve complex problems. In this article, we will explore the world of data science, its applications, required skills, workflow, challenges, future trends, and more.
What is Data Science?
At its core, data science is the practice of extracting knowledge from data using various scientific methods, algorithms, and tools. It involves collecting, cleaning, analyzing, and interpreting both structured and unstructured data to derive actionable insights. Data scientists utilize their expertise in statistics, programming, and machine learning to uncover hidden patterns, make predictions, and drive informed decision-making.
Applications of Data Science
Data science has permeated almost every industry, revolutionizing the way businesses operate and make strategic decisions. In healthcare, data science is being used to develop personalized treatment plans, predict disease outbreaks, and improve patient outcomes. In finance, data scientists are leveraging data to detect fraud, optimize investment portfolios, and assess creditworthiness. Marketing teams are harnessing the power of data science to target specific customer segments, optimize ad campaigns, and enhance customer experiences. These are just a few examples, and the applications of data science continue to expand across various sectors.
Skills Required for Data Scientists
To embark on a successful career in data science, one must possess a combination of technical skills, analytical mindset, and domain knowledge. Proficiency in programming languages like Python and R is essential for data manipulation, analysis, and model building. A solid foundation in statistics and mathematics helps in understanding the underlying principles of data science algorithms. Additionally, expertise in machine learning, data visualization, and database management are highly valued skills in the field.
Data Science Workflow
Data science projects typically follow a well-defined workflow that ensures a systematic approach to problem-solving. This workflow begins with problem identification, where the data scientist understands the business problem and defines clear objectives. The next step involves data collection, where relevant data is gathered from various sources. Once the data is collected, it undergoes a thorough cleaning and preprocessing phase to handle missing values, outliers, and inconsistencies. Exploratory data analysis techniques are then applied to gain insights and discover patterns within the data. The subsequent stages involve model building, evaluation, and deployment, where machine learning algorithms are trained, tested, and integrated into real-world systems.
Machine Learning and Data Science
Machine learning is a subset of data science that focuses on developing algorithms and models that can learn from data and make predictions or decisions. It plays a crucial role in data science projects, enabling the extraction of valuable insights from complex datasets. Supervised learning algorithms, such as linear regression and support vector machines, are used for predictive modeling, while unsupervised learning algorithms, like clustering and dimensionality reduction, help in discovering patterns and grouping similar data points. Reinforcement learning algorithms enable machines to learn through trial and error, which is particularly useful in fields like robotics and autonomous vehicles.
Challenges in Data Science
Despite its immense potential, data science is not without its challenges. One of the primary concerns is data quality, as incomplete or inaccurate data can lead to erroneous insights and unreliable predictions. Privacy and ethical considerations are also critical, especially with the increasing amount of personal and sensitive data being collected. Data governance practices, such as data anonymization and secure storage, are vital to protect individuals' privacy. Furthermore, the rapid pace of technological advancements and the ever-increasing volume of data pose additional challenges that data scientists must navigate.
Future Trends in Data Science
The field of data science is constantly evolving, and staying abreast of emerging trends is crucial for data scientists. Artificial intelligence (AI) is set to play a significant role, with advancements in deep learning enabling machines to understand and interpret complex data, such as images and natural language. Natural language processing (NLP) is another area garnering attention, as it allows machines to comprehend and generate human language, leading to applications like chatbots and language translation. As data continues to grow exponentially, the ability to extract meaningful insights from unstructured data, such as text and images, will become increasingly important.
DS Conclusion
Data science has emerged as a game-changer in today's data-driven world. By leveraging the power of data, businesses and organizations can gain valuable insights, make informed decisions, and stay competitive. The demand for skilled data scientists continues to rise, presenting exciting opportunities for those looking to enter this field. As data science evolves, it will unlock new possibilities, transform industries, and shape the future of technology.
DS FAQs
FAQ 1: What programming languages should I learn for a career in data science?
To excel in data science, it is recommended to learn programming languages like Python and R. These languages offer extensive libraries and tools specifically designed for data manipulation, analysis, and machine learning.
FAQ 2: Can data science be applied to non-technical fields?
Absolutely! Data science is applicable to a wide range of domains, including non-technical fields like healthcare, finance, marketing, and social sciences. The ability to extract insights from data is valuable across various industries.
FAQ 3: How long does it take to become a proficient data scientist?
The time required to become proficient in data science varies depending on individual circumstances and prior knowledge. It generally takes several months to a few years of dedicated learning and hands-on experience to develop the necessary skills.
FAQ 4: What is the average salary of a data scientist?
Data scientists are in high demand, and their salaries reflect this. The average salary of a data scientist varies depending on factors such as location, industry, and experience. However, it is generally considered a lucrative career path.
FAQ 5: Are there any online courses or resources to learn data science?
Yes, there are numerous online courses and resources available to learn data science. Platforms like Coursera, edX, and DataCamp offer a wide range of courses and tutorials catered to different skill levels. Additionally, there are open-source tools and libraries, such as scikit-learn and TensorFlow, that provide extensive documentation and examples for learning data science.
DS is a diploma level course. Siddha, which means the study of life, is an old and tried and true therapeutic study of India, utilized as an option restorative framework for keeping up the mental, physical, and otherworldly soundness of individuals. In the wake of finishing this one year certification in Ayurveda, understudies can construct dynamic professions in the fields of clinical practice, scholastics, facility the board and organization, tranquilize assembling, and research, as ayurvedic health experts, fragrance specialists, ayurvedic professionals/specialists, knead advisors, botanists, fitness coaches, yoga educators, collaborators to medication producing, inquire about partners in Ayurveda, instructors in Ayurveda in schools, and so forth.
Job Profile: